Megan Stevenson Releases Groundbreaking Paper on Judges’ Use of Algorithms in Sentencing Decisions
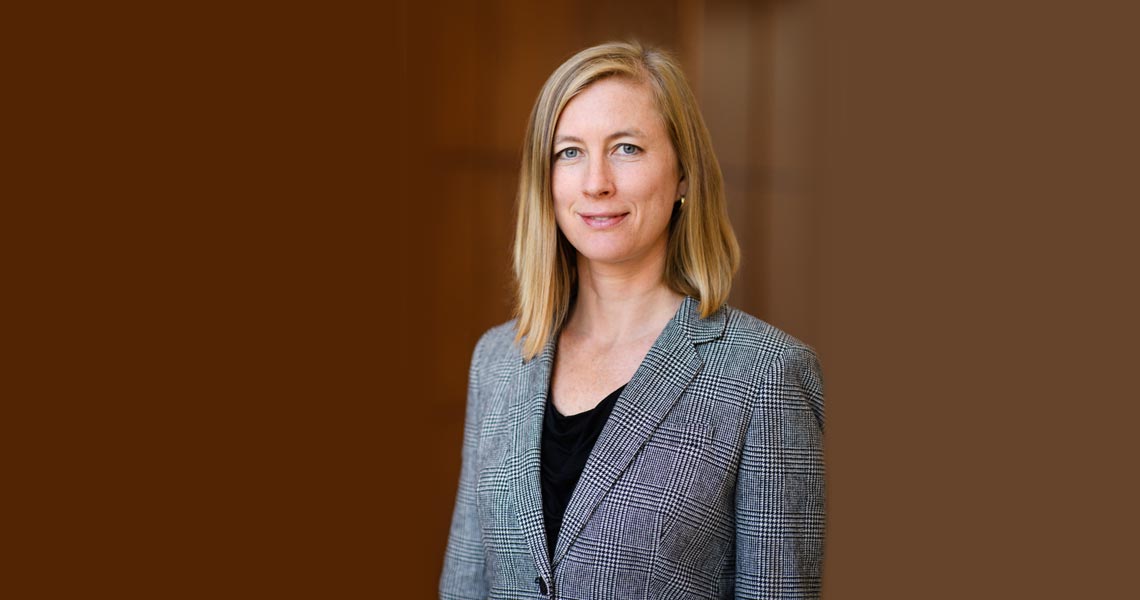
By Andrew Van Dam
We tend to assume the near-term future of automation will be built on man-machine partnerships. Our robot sidekicks will compensate for the squishy inefficiencies of the human brain, while human judgment will sand down their cold, mechanical edges.
But what if the partnership, especially in the early stages, instead accentuates the flaws of both? For example, a formula designed to reduce prison populations in Virginia led some judges to impose harsher sentences for young or black defendants, and more lenient ones for rapists.
In an age when artificial intelligence is widely expected to eat what’s left of the world, simple sentencing algorithms are a preview of the economy’s tool-assisted future. A working paper released Tuesday by Megan Stevenson of George Mason University and Jennifer Doleac of Texas A&M provides one of the first examinations of the unintended consequences that arise when algorithms and humans team up in the wild.
The algorithms are intended to remove some of the guesswork from judges’ sentencing decisions by assigning a simple risk score to defendants. In Virginia, the score included data such as offense type, age, prior convictions and employment status. Larceny scores higher than drug offenses, men score higher than women, and unmarried folks score higher than their married peers. (Marriage and employment were removed from the score in 2013.) Similar algorithms are now used in 28 states (and parts of seven more). In Virginia, they were adopted statewide in 2002 to help keep prison populations down after discretionary parole was abolished. Stevenson and Doleac’s analysis relies on tens of thousands of felony convictions, with a particular focus on the period between 2000 and 2004.
Read more at The Washington Post